
Other research projects
Project 1 | Alleviating drought stress in rice: a nanoparticle mediated approach
Drought is a critical reoccurring global abiotic stress that negatively impacts crop quality and quantity. Urbanization, and domestic water use place further pressure on agriculture by competition for arable land and water. In India, droughts occur frequently, resulting in water shortages and economic crises for rural people, eventually leading to food insecurity. Burgeoning population in the country has led to increased demand for water and other natural resources, exacerbating the impact of droughts. Thus, in economic terms, yield loss from drought has severe ramifications not only for farming households, but also for the entire agrarian communities, whole regions, and even nations.
In recent years, scientists have shown the potency of nanomaterials in different agricultural sectors and have successively developed nanotechnology to enhancing crop productivity at low cost and energy usages. Nanomaterials are found to improve seed germination, germination rate, augment photosynthetic activities, show significant effects on various agronomic traits and provide protection against pest and diseases, to name a few. Though nanotechnologies were greatly utilized in various sections in agriculture, however, till date, research on the application of nanomaterials in decreasing drought stress in rice has never been carried out systematically.
The overarching goal of the proposed research proposal is to engineer nanoparticles to alleviate drought stress in rice plants by boosting their inherent defence mechanisms, leading towards the development of related nanotechnology. Few nanomaterials like ZnO, SiO2 etc., used previously as micro-nutrient, were found to impart drought resilience in wheat, sorghum and other crops. Mechanistically, reactive oxygen species (ROS) scavenging and enzymatic activities were proposed to play important roles in drought amelioration in plants. Given that rice is the most important cereal crop of our country and is predominantly cultivated as a rainfed crop during monsoon, such a study could generate valuable information in reducing the yield loss due to drought stress as a result of rainfall deficiency. Following is the schematic representation of the different project activities, institute wise responsibilities and decision tree to be undertaken during the implementation of the project
Future project | Machine learning for image-based identification of nematode genera
Accurate species identification is the basis for all aspects of taxonomic research and is an important component of workflows in any biological research. Many activities, such as studying the biodiversity richness of a region, monitoring populations of endangered species, determining the impact of climate change on species distribution and nematode control actions depend on accurate identification skills. Automating the task and making it feasible for non-experts is highly desirable, especially considering the continuous loss of biodiversity and lack of experienced taxonomists.
Advances in machine learning also referred to as deep learning or artificial intelligence (AI), have opened a new avenue for nematode identification and quantification based on image analysis. The technique is especially suitable for handling large numbers of samples as well as for detecting rare species and objects. Previous studies show that AI can play a big role in the detection, quantification as well as classification of nematodes. As such, it will help address some of the limitations associated with the traditional morphology-based classification including the dwindling number of taxonomists, subjective decision making, and provide fast and accurate identification. Limitations arising from shared morphological features between taxa would likely remain, but there is the possibility that machine learning will be able to elucidate unique characters discriminating nematodes that have been undetected even by the trained human eye. Here we propose a machine learning framework for image-based identification of nematode genera and biodiversity assessment. Such an architecture could help in circumventing the limitations associated with the scarcity of highly qualified taxonomists and enables objective decision making, coupled with fast and accurate identification. The following figure shows the scheme of the proposed research.
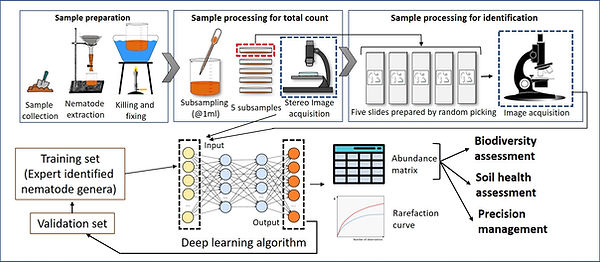